Dr. John Jolliffe is Project Manager for NFDI4Chem at the Johannes Gutenberg University in Mainz, Germany. In this interview with Dr. Vera Koester for ChemistryViews, he discusses effective research data management for chemists, the advantages of electronic lab notebooks, their impact on scientific progress, and the fears scientists may have.
What is NFDI4Chem in three sentences?
NFDI4Chem is a DFG-funded, non-commercial consortium run by the community for the community.
We develop and maintain a national research data infrastructure for the chemistry research community in Germany.
We support scientists in their efforts to collect, store, process, analyze, publish, and reuse research data in chemistry.
Who are we?
It’s not like an organization was created out of thin air and a bunch of people were hired. Existing research data experts came together and are now creating an infrastructure that none of them could create on their own.
NFDI4Chem is supported by a community of researchers, data scientists, and experts in chemistry and data management, working together to develop and maintain the infrastructure and services it offers. We have the learning societies on board, for example, the German Chemical Society (GDCh), the German Pharmaceutical Society (DPhG), the German Bunsen Society (DBG), we have people from research data infrastructure, e.g., institutions such as the KIT, FIZ Karlsruhe, or the TIB Hannover, and, most importantly, we have active researchers, who are in academia doing research and have been doing research in a reasonably FAIR way and are sharing their best practices. So it’s a real broad spectrum of expertise that’s coming together here to drive the future forward.
Why are you doing this and what is the main advantage for the researchers?
Currently, researchers, particularly in specific chemistry domains, conduct and publish their research without consistently including the corresponding data in their publications. They think they might be publishing their data but it’s more representations of data. So a supporting information document gets generated, and all the spectra are there in the forms of PDFs or JPEGs but you can’t really interact with that data. So it doesn’t really make them reusable in any sense.
Especially with the advent of machine learning tools that need data to be trained on, we need our research data to be both human-readable and machine-readable. But doing that is not that straightforward. For data to be machine-readable what helps is making them FAIR. So according to the FAIR principles, findable, accessible, interoperable, reusable.
Why is FAIR data important? And what does FAIR stand for?
Data is the currency of science. All scientific results are based on data. Data is what makes science replicable, gives credibility to science, makes it reproducible. However, the quality of data can vary widely. Researchers should be aware of data management practices, metadata standards, data-sharing policies, and ethical considerations related to data collection, storage, and sharing.
FAIR is an acronym for “findable, accessible, interoperable, reusable”. It doesn’t just describe the data itself but also the metadata. So the metadata is the data about the data that puts the data in a semantic context such as how it links to an experiment, and how it links to other types of data.
We have all the aspects of FAIR described in depth in our NFDI4Chem knowledge base explaining the principles from a chemist’s point of view. It’s quite a long article because there are a lot of definitions of what “FAIR” actually means. To give you a short introduction:
F, findable, means that the data and metadata can be found by a persistent identifier for example. So if a dataset has a DOI, it means you can find it.
A, accessible, means that the data actually exists somewhere where you can find it. A good example of this is an open-access repository that assigns DOIs to all of its datasets.
I, interoperable, is about the format that the data is in. If you’re doing analytical methods with proprietary software and you publish the output of the proprietary software, it’s only usable by those who have that proprietary software, and if that proprietary software becomes obsolete at some point, the data may be useless. So it’s important to have data in, let’s say, persistent formats. These are called open formats, and there are also open formats that are defined as standards, for example, JCAMP-DX, which is an IUPAC standard for spectral type of data.
R stands for reusable. So who can use the data? You can apply licences to data, for example, CC licences. It is important to stress that open does not mean FAIR. Quite often there’s a misconception that if you make your data FAIR, it means everybody has access to it. There’s a Venn diagram. You have open data that is FAIR, you have FAIR data that is open, but you also have FAIR data that’s not open, and vice versa. You can actually have FAIR data that is subject to an access embargo.
So for us, yes, we are vehement supporters of open data, but first and foremost our priority is that the data is FAIR, because if open data is not FAIR, then it’s not much use.
So if I’m a researcher, how do I find out what I need to do, how to store data, and which format to use?
It’s obviously not something you do overnight. These are skills that aren’t taught at an undergraduate level and so a lot of researchers are finding themselves confronted with the acronym FAIR and don’t really know what to do. In a recent survey we did, over 80 % of people said they’d like to see this as part of the undergraduate curriculum. Together with the GDCh, we’ve actually made a recommendation on how to get these digital literacy skills into the curriculum, but until that happens, it’s kind of our job to fill the gap a little bit as well.
NFDI4Chem has different means by which researchers can acquire this knowledge. We have very low-level entry points, like a YouTube channel where we have explainer videos for different things. We have a knowledge base that is kind of like a Wikipedia for research data management but from a chemistry point of view. There are knowledge bases out there for research data management, but they’re very interdisciplinary, and sometimes the focus is on something that’s not that relevant to a chemist.
There are different levels of depth in this knowledge base, so you can read very basic things such as what are the FAIR principles, what is an electronic lab notebook, but then you can also read more in-depth articles such as I’m a synthetic chemist, what are the data types that I use and what are the open format equivalents.
You can actually enter the knowledge base through different entry points, be it your role, are you a researcher, a PI, or a data steward, you can enter it through a domain, so are you a physical chemist, an inorganic chemist, a synthetic chemist, but then also by specific topics like data handling.
What we’re really proud of is that we have a series of workshops. We have one called “FAIR Research Data Management Basics for Chemists“. We started out alternating between an online and an institutional workshop, but now we’ve had so many requests from institutions that we’re focusing more on doing these workshops at institutions. We go to universities and take them through the basics of research data management in a two-day course but from a chemist’s point of view. It’s kind of an introductory course on what’s what.
So every professor today has to or should have this kind of knowledge. But what do you say to somebody who has a lot of other things to do and maybe thinks, I’ve been doing this all my life, why should I switch to this new stuff? Isn’t it a lot of extra work for no real effort?
It depends on how you do it. It can actually save you a lot of work if you use the right tools. And nobody works FAIR overnight; FAIR is not an absolute, FAIR is a spectrum. And there are little things you can do. For example, you can introduce naming conventions for your folders in your research group.
But the reality is that to work in a FAIR way in chemistry, if you take existing workflows, paper lab notebooks and saving things to folders and everything, if you then want to publish that data in a FAIR way, it is incredibly time-consuming because you have to do a lot of steps manually, such as assigning the appropriate metadata, converting to open formats so that it is classified as FAIR when you publish it to a repository. So to actually not invest too much time, you need tools to help you work FAIR. And a really good tool for that is electronic lab notebooks. An ELN can really help you to do the FAIR aspects automatically. So right from the beginning, it assigns the metadata in a standardized way. Everything is described according to the control of the capabilities that are set by ontologies, and it structures the data in a standardized way so that when you want to publish things, the ELN has already done all the formatting for you so that you don’t spend any more time when you publish it.
These ELNs can save you a lot of time on other things as well because they have quality control mechanisms built in.
We will come back to ELNs in a moment, but first I would like to know if publishers and funding organizations are pushing or supporting this. Are you working with them?
Yes, absolutely. Especially with publishers, we have a great format called Editors4Chem. It is an open forum between many publishers. At the last workshop we had in November, I think we had over 20 editors show up. It is really a discussion about how we can move to a future where we work in a reasonably FAIR way.
I think for publishers their main motivation for FAIR data is also the reproducibility crisis because it is very hard to commit scientific fraud if you have to publish data as well.
The German Research Foundation (DFG) is also very interested in FAIR data.
And at the international level?
Internationally, we are less involved with funders, but we are quite heavily involved with IUPAC. There is no point in setting a standard for Germany. The process of standardization is incredibly important. IUPAC has a fantastic track record of setting standards in all sorts of different areas. We are really grateful to be involved in their working groups.
Back to electronic notebooks. What are ELNs and why is this a topic for NFDI4Chem?
Electronic lab notebooks (ELNs) are digital tools that replace traditional paper lab notebooks. They enable scientists to record and manage experimental data, observations, and research notes electronically, offering features for data organization, collaboration, and searchability.
The ELN is relevant to NFDI4Chem because it provides a platform for sharing information, updates, and resources related to the initiative, helping researchers stay informed about developments in data management and collaboration opportunities within the chemistry community.
For someone who has no idea about ELNs, how should that person start to find out what kind of products are out there, how to find one that suits you, how much money you have to spend …
So these are really important questions to consider, and which ELN is the right ELN for you is not something that should be taken lightly. It should be a thorough evaluation process. You do not have to reinvent the wheel, there are some fantastic guidelines out there from ZBMed and they have also developed a fantastic tool called the “ELN Finder“. It is a repository of different ELNs and it can really help you choose an ELN.
Basically, in a nutshell, you have to define for yourself what you want from an ELN. Should it be open source or should it be commercial, do I want something that is software as a service or something that is hosted at my institution? If you are working with industrial collaborators, you may be more interested in an ELN where the data is hosted on-site rather than off-site. What are the costs? What are the staffing costs? How much effort will it take to maintain this ELN? And what do I want to do with the ELN? This is a really, really important question.
A lot of people think, okay, if I use an ELN, I’m going to be FAIR. That is not the case. A lot of commercial ELNs actually come from an industrial context and are focused on keeping data organized within a company. But they’re not very good at actually getting the data out of there and publishing it. It makes sense from an industrial point of view. From an academic point of view, that is not necessarily what you want to do.
It’s a good idea to narrow it down to about three or four different ELNs and then do a thorough field test in the lab. So, for a couple of months, divide your lab into a couple of groups that are testing the ELN in their day-to-day research, not just playing around with it. And then you share your experiences, and then at the end you decide on an ELN; and then there’s also no one-size-fits-all way to implement it.
Some research groups find that it works quite well, that you make a cut, that every new person that comes in from a certain point of time has to use the ELN. At some institutions, for example at the University of Aachen, they actually have ELNs in the undergraduate curriculum now, so the teaching labs use an ELN and students already get familiar with the software.
So sometimes it is also the university that is driving this?
It really depends on the university and it’s a fascinating landscape.
Some universities actually say this is the ELN you should use, which is not very helpful because there’s no one ELN that’s going to make everyone equally happy. So if you force an entire chemistry department to use one ELN, you’re going to make some people very happy and some people less happy, depending on what ELN is there, because there are sub-discipline specific ELNs, but there are also more generic ELNs.
Some sub-disciplines are not going to be as happy with the generic ELNs because they’re missing specific functionality. So, for example, a chemical reaction scheme should be drawn automatically in an interactive way by dropping in a sample and you don’t have to draw the reaction scheme manually every time. So for a synthetic
chemist, for example, a very generic ELN can be a bit frustrating because you have to do too much manually.
Do you need to have someone in your group who is a sort of technical specialist who can solve problems with the ELN, or is it all self-explanatory?
That’s a very excellent question because the way things often work in a research group is that you have some sort of job that gets passed down. So one Ph.D. student does it and they pass it on to another Ph.D. student.
For some ELNs that might work because they are simple enough or a lot of the stuff is maintained off-site. But some ELNs may require a little bit more maintenance and also someone who does the admin. In some academic groups that we know of, the academic councils or the lab managers do it. Some working groups are part of SFBs [collaborative research centres] and some SFBs have data stewards, some universities have research data departments that take care of these things.
There’s actually a lot of expertise at universities, but often people don’t necessarily realize that they have research data experts at the university.
In terms of collaboration, you mentioned SFB projects, is it easy to transfer data or collaborate between people using different ELNs?
To date, interoperability between ELNs is somewhat limited, especially if you have a very sub-domain specific ELN and you have a collection of data in there, experiments that you want to transfer to another ELN, that’s very difficult at the moment.
There are efforts to make these things interoperable, to find the common denominator, but as you can imagine that’s a process that doesn’t happen very quickly because all these systems were developed in parallel and each has its own entirely different software, database libraries, and finding a common denominator is not something that happens overnight.
That’s also why for Chemotion, the ELN that we’ve developed, we’re trying to make it as interdisciplinary as possible. Our ELN actually started off from a synthetic point of view. Once you get out of synthesis, the workflows are very different from workgroup to workgroup. So we’d have to develop hundreds of different user interfaces (UIs) to make all the researchers happy. So our approach is actually to give the researchers a toolkit to build their own UIs without requiring any programming knowledge.
Researchers naturally care a lot about their data, so they make sure that they can keep it away from people they don’t want to see it. Can you do that with ELNs?
Again, it depends on what kind of ELN model you’re using. If you’re using software as a service, you’re as secure as the vendor that’s providing it. If you’re using a locally hosted ELN, then the data is no less secure than it already is on some institutional server where the analytical data is anyway.
In our ELN, when you create an experiment, only you can see it, and only someone else can see it if you choose to share it with them. Even the administrators can’t see it.
Actually, ELNs can be an incredibly powerful tool to work more ethically because ELNs can have audit trails in them and actually in certain scientific fields you are required to use an ELN with an audit trail so you can actually see who has changed what. For example, somebody would do an experiment, and in retrospect, it turns out that they made a bad mistake, or committed fraud, and then they would go back and delete the evidence. With an audit trail, you can’t do that.
What fascinates you most about ELNs, personally and as a consortium?
I think for us, it’s really about seeing the potential of what you can do with data. If you look at the recent advent of machine learning, the progress that’s happening is incredible. The workflow of the future is going to have a lot of these software tools like retro synthetic tools integrated into an ELN. If you want to make this molecule, you have a plugin that already suggests this might be the way it works. It’s linked to databases that tell you it’s going to cost this much. So everything can be so much faster.
At the end of the day, these tools reduce the number of experiments and, therefore, save resources. That’s exactly the mission of NFDI4Chem. We are doing this for the betterment of science and the advancement of science.
For me personally, this is something that I really believe in. It is really fun to be part of a project that is doing something for the sake of science.
If you had known all of this about FAIR data and ELNs during your Ph.D., would things have been different?
So much different. It’s like I see things in the ELN now and sometimes I wish I had had that. So we have a tool in our ELN that can automatically generate a supporting information document … and then I think “it would have taken me a month to write that manually”. It really automates a lot of things, it reduces the amount of copy and paste errors that you make, the human errors, it does quality control, it helps you review things. It’s also a great way to keep track, to keep you characterizing compounds as you go along. But it’s important to realize that an ELN is first and foremost linking experimental data to an experimental description.
In the past, I would take over a project and then I would look for my predecessor’s data. First I have to find his lab books. What room are the lab books in, what shelf are they on, what book is it, what page? And then once I’m on the page, I’d find the experiment number, and then the next hunt is to go to the service and find the things, and sometimes you don’t even find the data.
I actually know of cases where a researcher could not find their predecessors’ data. They had to redo a whole synthesis, and then it turned out that the assignment of the compound was wrong, and so everything was wrong and basically, seven months of work went down the drain. These are things that can be avoided with an ELN. It also saves money. Think about seven months of public money that could have been saved if the data had been documented properly.
… and your life.
Yes, that too. I feel very sorry for this person.
For anyone who has not done anything in this direction, what would be the first best tips to get started?
First of all, you have to want to learn.
Take baby steps. You do not have to do 100 % right from the start. See what you can do right now and it is really good to put yourself in someone else’s shoes. Let’s say someone else is trying to reuse my data, so what should I do?
Learn from others. Look around your research group and see how things are done. Look in your department to see if there are experts, such as research data management experts.
And then, if you have questions, we are here to help. We have a help desk where you can ask all those questions. We have the training opportunities that we mentioned. We are there to really support this cultural change. You’re not alone, don’t think it’s too much. Ask, that’s what we’re here for.
Thank you very much for the interview.
John Jolliffe studied chemistry at the University of Newcastle, UK, and received his Ph.D. from the University of Oxford, UK, in 2016. After postdoctoral positions at the University of Oxford and the Technical University of Munich, Germany, he was a Project Manager at Midas Pharma, Ingelheim, Germany, from 2019 to 2020, and has been a Research Associate at the Johannes Gutenberg University of Mainz, Germany, and Project Manager for NFDI4Chem since 2021.
- NFDI4Chem website
Also of Interest
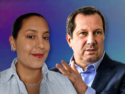
A. Förster and S. Espinoza, DECHEMA, discuss the goals and benefits of NFDI4Cat and the transformative impact of FAIR data sharing on catalysis research
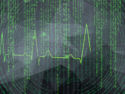
NFDI4Chem is an initiative to build a FAIR infrastructure for research data management in chemistry and offers free tools, resources, and trainings
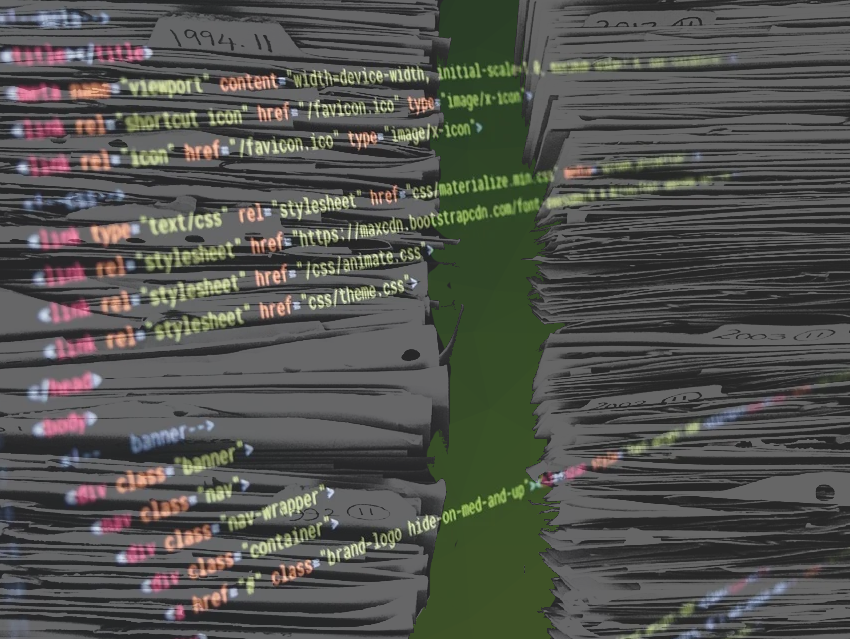
A survey among German scientists shows that tools are often still missing to collect, store, and share data
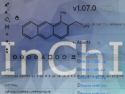
Enhances transparency, collaboration, and reliability, supports future expansions, and promotes broader adoption of the International Chemical Identifier for linking chemical information across web sources
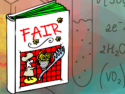
IUPAC launched interactive guide for digital chemical data – The FAIR Chemistry Cookbook
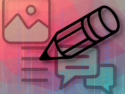
A collection of useful resources for chemists and other scientists that help with text editing, citations, image generation
Thanks