The Nobel Prize in Physics 2024 has been awarded to
- John Joseph Hopfield, Princeton University, NJ, USA,
- Geoffrey E. Hinton, University of Toronto, Canada
for “foundational discoveries and inventions that enable machine learning with artificial neural networks” [1].
John Hopfield developed an associative memory system that enables the storage and reconstruction of data patterns, while Geoffrey Hinton created a method for autonomously detecting specific elements in images. Their groundbreaking work has significantly advanced the field of computing, influencing various applications, e.g., in artificial intelligence.
1 Research
Hopfield and Hinton have developed methods that are the foundation of powerful machine learning (ML) methods.
Machine learning using artificial neural networks often serves as the basis of artificial intelligence technology. Neural networks were originally inspired by the structure of the brain. In an artificial neural network, the brain’s neurons are represented by “nodes” that have different values. These nodes influence each other through connections that can be likened to synapses. When the network is trained, the connections between nodes that are active at the same time get stronger, otherwise, they get weaker.
1.1 The Hopfield Network
John Hopfield invented a network that can store and recreate patterns, known as an “associative memory” [2]. We can imagine the nodes as pixels in an image. The Hopfield network uses physics to describe a material’s characteristics based on its atomic spin. For example, the nodes can have values of 0 or 1 to represent the pixels in a black-and-white picture. The network as a whole is described in terms of the energy of the physical spin system. It is trained by finding values for the connections between the nodes so that the saved images have the lowest possible energy.
When the Hopfield network is fed a distorted or incomplete image, it methodically works through the nodes and updates their values so the network’s energy is minimized. The network, thus, works stepwise to find the saved image that is most like the imperfect one it was fed with. The Hopfeld network can be used, e.g., to recreate data that contains noise or has been partially erased.
1.2 The Boltzmann Machine
Geoffrey Hinton used the Hopfield network as the foundation for a new network that uses a different method, called the Boltzmann machine [3,4]. Unlike the Hopfield model, the Boltzmann machine focuses on statistical distributions of patterns rather than individual patterns. This network can learn to recognize characteristic elements in a given type of data. Hinton used tools from statistical physics.
The Boltzmann machine is commonly used with two different types of nodes. Information is fed to one group, which is called visible nodes. The other group of nodes forms a hidden layer. The hidden nodes’ values and connections also contribute to the energy of the network as a whole. They are included to enable the modeling of more general probability distributions.
The machine is run by applying a rule to update the values of the nodes one at a time. Eventually, the machine will enter a state in which the nodes’ pattern can change, but the properties of the network as a whole remain the same. Each possible pattern will then have a specific probability that is determined by the network’s energy according to a Boltzmann distribution. In statistical physics, the Boltzmann distribution is a probability distribution that gives the probability that a system will be in a certain state as a function of that state’s energy and the temperature of the system.
The Boltzmann machine can learn by being given examples. It is trained by updating the values in the network’s connections so that the example patterns, which were fed to the visible nodes during training, have the highest possible probability of occurring when the machine is run. A trained Boltzmann machine can recognize familiar traits in information it has not previously seen. It can be used, e.g., to classify images or create new examples of the type of pattern on which it was trained. Hinton has built upon this work and helped to initiate the current explosive development of machine learning.
2 Laureates
John Joseph Hopfield, born in Chicago, IL, USA, in 1933, studied at Swarthmore College, PA, USA, and earned a Ph.D. in physics from Cornell University, Ithaca, NY, USA, in 1958, supervised by Albert Overhauser. After two years in the theory group at Bell Laboratories, he subsequently became a faculty member at the University of California, Berkeley, USA, Princeton University, and the California Institute of Technology (Caltech), Pasadena, CA, USA, and again at Princeton University, where he currently is the Howard A. Prior Professor of Molecular Biology, Emeritus.
In 1986, he co-founded the Computation and Neural Systems PhD program at Caltech.
John J. Hopfield was awarded many honors, including the Boltzmann Medal alongside Deepak Dhar in 2022, the Albert Einstein World Award of Science in 2005, the Dirac Medal of the International Centre for Theoretical Physics (ICTP) in 2001, and the Golden Plate Award of the American Academy of Achievement in 1985. He was elected to the U.S. National Academy of Sciences in 1973, the American Academy of Arts and Sciences in 1975, and the American Philosophical Society in 1988.
Geoffrey E. Hinton, born in London, UK, in 1947, studied at Clifton College, Bristol, UK, and King’s College, Cambridge, UK, focusing on various subjects, including natural sciences, history of art, and philosophy, and graduated in 1970 with a Bachelor of Arts in experimental psychology. He earned his Ph.D. from the University of Edinburgh, UK, in 1978 for research in artificial intelligence, supervised by Christopher Longuet-Higgins. After positions at the University of Sussex, UK, the University of California, San Diego, USA, and Carnegie Mellon University, Pittsburgh, PA, USA, he became the founding director of the Gatsby Charitable Foundation Computational Neuroscience Unit at University College London, UK. Currently, he is a professor in the computer science department at the University of Toronto, Canada.
Geoffrey Hinton holds a Canada Research Chair (a title given to certain Canadian university research professors by the Canada Research Chairs Program) in Machine Learning and, as of June 2024, serves as an advisor for the Learning in Machines & Brains program at the Canadian Institute for Advanced Research. He joined Google in 2013 after his company, DNNresearch Inc., was acquired, while continuing his academic research.
Geoffrey Hinton was elected a Fellow of the Royal Society (FRS) in 1998 and of the Association for Computing Machinery (ACM) in 2023. He became the first recipient of the Rumelhart Prize in 2001. He was awarded the 2011 Herzberg Canada Gold Medal for Science and Engineering, the Canada Council Killam Prize in Engineering in 2012, the Turing Award in 2018 along with Yann LeCun and Yoshua Bengio for their groundbreaking work on deep neural networks. That same year, he was named a Companion of the Order of Canada.
References
[1] Website of the The Nobel Foundation nobelprize.org
[2] J. J. Hopfield, Neural networks and physical systems with emergent collective computational abilities, Proc. Natl. Acad. Sci. USA 1982, 79, 2554–2558. https:/doi.org/10.1073/pnas.79.8.2554
[3] S. E. Fahlman, G. E. Hinton, T. J. Sejnowski, Massively Parallel Architectures for Al: NETL, Thistle, and Boltzmann Machines, Proc. AAAI-83 Conf. 1983, 109–113.
[4] D. H. Ackley, G .E. Hinton, T. J. Sejnowski, A learning algorithm for boltzmann machines, Cogn. Sci. 1985, 9, 147–169. https://doi.org/10.1016/S0364-0213(85)80012-4
Selected Publications
Selected Publications by John J. Hopfield
- Dmitry Krotov, John J. Hopfield, Unsupervised learning by competing hidden units, PNAS 2019, 116(16), 7723–7731. https://doi.org/10.1073/pnas.1820458116
- John J. Hopfield, Understanding Emergent Dynamics: Using a Collective Activity Coordinate of a Neural Network to Recognize Time-Varying Patterns, Neural Computation 2015, 27(10), 2011–2038. https://doi.org/10.1162/NECO_a_00768
- Filip Ponulak, John J. Hopfield, Filip Ponulak, John J. Hopfield, Rapid, parallel path planning by propagating wavefronts of spiking neural activity, Front. Comput. Neurosci. 2013. https://doi.org/10.3389/fncom.2013.00098
- John J. Hopfield, Neurodynamics of mental exploration, PNAS 2009, 107(4), 1648–1653. https://doi.org/10.1073/pnas.0913991107
- David A. Markowitz, Forrest Collman, Carlos D. Brody, David W. Tank, Rate-specific synchrony: Using noisy oscillations to detect equally active neurons, PNAS 2008, 105(24), 8422–8427. https://doi.org/10.1073/pnas.0803183105
- J. J. Hopfield, Dynamics and neural network computation, Int. J. Quantum Chem. 1990. https://doi.org/10.1002/qua.560382461
Selected Publications by Geoffrey E. Hinton
- Alex Krizhevsky, Ilya Sutskever, Geoffrey E. Hinton, Net classification with deep convolutional neural networks, Commun. ACM 2017, 60, 84-90. https://doi.org/10.1145/3065386
- Nitish Srivastava, Geoffrey Hinton, Alex Krizhevsky, Ilya Sutskever, Ruslan Salakhutdinov, Dropout: A Simple Way to Prevent Neural Networks from Overfitting, Journal of Machine Learning Research 2014, 15, 1929−1958. https://jmlr.org/papers/volume15/srivastava14a/srivastava14a.pdf
- Laurens van der Maaten, Geoffrey Hinton, Visualizing Data using t-SNE, J. Mach. Learn. Res. 2008, 9, 2579–2605. https://www.jmlr.org/papers/volume9/vandermaaten08a/vandermaaten08a.pdf
- Geoffrey Hinton, Artificial Intelligence: Neural Networks, Van Nostrand’s Scientific Encyclopedia, Wiley, USA, 2005. https://doi.org/10.1002/0471743984.vse0673
- David S. Touretzky, Geoffrey E. Hinton, A Distributed Connectionist Production System, Cognitive Science 1988. https://doi.org/10.1207/s15516709cog1203_4
- Geoffrey E. Hinton, Models of human inference, Cognitive Science 1987. https://doi.org/10.1111/j.1467-8640.1987.tb00194.x
- David H. Ackley, Geoffrey E. Hinton, Terrence J. Sejnowski, A Learning Algorithm for Boltzmann Machines, Cognitive Science 1985. https://doi.org/10.1207/s15516709cog0901_7
Also of Interest
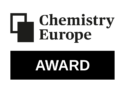
The 10.000 EUR award recognizes outstanding contributions to the field of theoretical/computational chemistry with an optional focus on AI in chemistry
- Nobel Prize in Physics 2023,
ChemistryViews 2023.
Pierre Agostini, Ferenc Krausz, and Anne L’Huillier were honored for experimental methods that generate attosecond pulses of light for the study of electron dynamics in matter - Who’s Next? Nobel Prize in Chemistry 2024,
ChemistryViews 2024.
Make your predictions for the 2024 Nobel Prize in Chemistry - Collection: Nobel Prize in Chemistry
Collection of interviews with Nobel Laureates and Nobel Prize quizzes