The Nobel Prize in Chemistry 2024 has been awarded to
- David Baker, University of Washington, Seattle, WA, USA,
for “computational protein design”, and the other half jointly to
- Demis Hassabis, Google DeepMind, London, UK, and
- John M. Jumper, Google DeepMind, London, UK
for “protein structure prediction” [1].
The work of the three researchers has answered a long-standing question in biology: Can we predict how a protein folds from its amino acid sequence? Their computational methods offer highly accurate models of protein folding.
1 Research
1.1 Protein Structure Prediction
In proteins, amino acids are linked together to form linear peptides that fold to create a three-dimensional structure, which is key for the protein’s function. Predicting protein structures from amino acid sequences is usually notoriously difficult.
In 2020, Demis Hassabis and John Jumper presented an artificial intelligence (AI) model called AlphaFold2 [2]. With its help, they have been able to predict the structure of almost all of the 200 million known proteins. Since their breakthrough, AlphaFold2 has been used by more than two million people from 190 countries.
1.1.1 From Chess to Proteins
Demis Hassabis, who started playing chess at the age of four and achieved master level as a 13-year-old, started a career as a programmer and game developer in his teen years. He then began exploring artificial intelligence and neuroscience, with the latter allowing him to develop better neural networks for AI. In 2010, he co-founded DeepMind, a company that developed AI models for popular board games and was sold to Google in 2014. DeepMind achieved a breakthrough in AI by beating the champion player of the board game Go [3].
Hassisbis then tackled the problem of protein folding, developing the AI model AlphaFold [4]. The model achieved nearly 60 % accuracy in a prominent protein structure prediction competition (“Critical Assessment of Protein Structure Prediction”, or CASP), which was sufficient to win, but there was still progress to be made.
1.1.2 Jumping in with Improvements
John Jumper first studied physics and mathematics, and he realized that knowledge of physics could help solve medical problems while working with supercomputers to simulate proteins and their dynamics. In 2011, he began his doctorate in theoretical physics.
To save computer capacity, he started developing simpler and more ingenious methods for simulating protein dynamics. In 2017, after completing his doctorate, he heard rumors that DeepMind had started to predict protein structures. He had ideas about how to improve AlphaFold and joined the team. Jumper and Hassabis co-led the work that fundamentally reformed the AI model.
The new version of the model, called AlphaFold2, was influenced by Jumper’s knowledge of proteins. The team also started to use the innovation behind the recent enormous breakthrough in AI: neural networks called transformers.
These models can find patterns in enormous amounts of data in a more flexible manner than previously possible, and efficiently determine where the focus should be to achieve a particular goal. The team trained AlphaFold2 on the vast information contained in databases of all known protein structures and amino acid sequences, and the new AI architecture started delivering good results.
In 2020, when the organizers of the CASP competition evaluated the results, they understood that the arguably biggest problem in biochemistry was solved. In most cases, AlphaFold2 performed almost as well as X-ray crystallography at determining protein structures!
1.2 Computational Protein Design
Proteins are generally built from the 20 natural amino acids, which can be combined to form an endless amount of different peptides and proteins. In 2003, David Baker used computational methods to design a new protein that was unlike any previously known one. Since then, his work has produced many new protein creations, including proteins that can be used in pharmaceuticals, vaccines, nanomaterials, and sensors.
1.2.1 Rosetta
David Baker first studied philosophy and social science. However, he took a course in evolutionary biology which led him to change directions. He began to explore cell biology and became fascinated by protein structures. He worked on protein folding, and, at the end of the 1990s, he began to develop software that could predict protein structures: Rosetta [5]. The software was used in the CASP competition in 1998 and performed well.
The success of Rosetta led to a new idea: using the software in reverse. Instead of entering amino acid sequences in Rosetta and getting protein structures out, researchers might be able to enter a desired protein structure and obtain suggestions for its amino acid sequence, which would allow them to create entirely new proteins.
1.2.2 New Proteins Created From Scratch
Protein design allows researchers to create tailored proteins with new or improved functions. Often, scientists “tweak” existing proteins, so they can, for example, serve as tools in the chemical industry or break down hazardous substances. However, the range of natural proteins is limited, and creating proteins “from scratch” (de novo design) can improve the potential for obtaining ones with entirely new functions.
Baker designed a protein with an entirely new structure and then had Rosetta compute which type of amino acid sequence could result in the desired protein. To do this, Rosetta searched a database of all known protein structures and looked for short fragments of proteins that had similarities with the desired structure. Using a fundamental knowledge of proteins’ energy landscape, Rosetta then optimized these fragments and proposed an amino acid sequence.
Baker introduced the gene for the proposed amino acid sequence in bacteria that produced the desired protein, and then he determined the protein structure using X-ray crystallography. It turned out that Rosetta really could construct proteins. The protein that Baker had developed, Top7, had almost exactly the desired structure, which did not exist in nature [6].
Laureates
David Baker, born in Seattle, Washington, USA, on October 6, 1962, studied at Harvard University, Cambridge, MA, USA, and received his Ph.D. in biochemistry in 1989 from the University of California, Berkeley, USA, in the laboratory of Randy Schekman, where he focused on protein transport and trafficking in yeast. After postdoctoral work in biophysics with David Agard at the University of California, San Francisco, USA, he joined the faculty of the Department of Biochemistry at the University of Washington School of Medicine in 1993 and became a Howard Hughes Medical Institute Investigator in 2000. Currently, Baker is Head of the Institute for Protein Design and the Henrietta and Aubrey Davis Endowed Professor of Biochemistry at the University of Washington.
Among many other honors, David Baker received the Feynman Prize in Nanotechnology in 2004, the Sackler International Prize in Biophysics in 2008, the Breakthrough Prize in Life Sciences in 2021, and the Wiley Prize in Biomedical Sciences in 2023. He was elected a Fellow of the American Academy of Arts and Sciences in 2009.
Demis Hassabis, born in London, UK, on July 27, 1976, studied computer science at the University of Cambridge, UK. He worked as a programmer and game designer before receiving his Ph.D. in cognitive neuroscience from University College London (UCL), UK, in 2009. Hassabis showed remarkable talent for chess from the age of four, captained many England junior teams.
Demis Hassabis is the CEO and co-founder of DeepMind, a machine learning AI startup that was founded in London in 2010 together with Shane Legg and Mustafa Suleyman. In 2014, Google purchased DeepMind. DeepMind’s tool AlphaFold aims to accurately predict protein structures.
Demis Hassabis is a Fellow of the Royal Society and has won numerous awards for his work on AlphaFold, including the Breakthrough Prize, the Wiley Prize in Biomedical Sciences, the Canada Gairdner International Award, and the Lasker Award, all in 2023. In 2017, he was included in the Time 100 list of the world’s most influential people. He was knighted for services to AI in 2024.
John M. Jumper, born in the USA, studied at the University of Chicago, USA, where he received his Ph.D. in 2017 for research on the use of machine learning to simulate protein folding and dynamics, co-supervised by Tobin R. Sosnick and Karl Freed. He also studied physics at the University of Cambridge, UK, where he was a Marshall Scholar, and physics and mathematics at the Vanderbilt University, Nashville, TN, USA. He is currently a senior research scientist at DeepMind Technologies, London, UK.
John Jumper has won numerous awards, including the Wiley Prize in Biomedical Sciences in 2023. The journal Nature named Jumper one of the ten “people who matter” in science in its annual list of Nature’s 10 in 2021.
References
[1] Website of the Nobel Foundation nobelprize.org
[2] J. Jumper, R. Evans, A. Pritzel, T. Green, M. Figurnov, O. Ronneberger, K. Tunyasuvunakool, R. Bates, A. Zidek, A. Potapenko, A. Bridgland, C. Meyer, S. A. A. Kohl, A. J. Ballard, A. Cowie, B. Romera-Paredes, S. Nikolov, R. Jain, J. Adler, T. Back, S. Petersen, D. Reiman, E. Clancy, M. Zielinski, M. Steinegger, M. Pacholska,T. Berghammer, S. Bodenstein, D. Silver, O. Vinyals, A. W. Senior, K. Kavukcuoglu, P. Kohli, D. Hassabis, Highly accurate protein structure prediction with AlphaFold, Nature 2021, 596, 583-589. https://doi.org/10.1038/s41586-021-03828-1
[3] S. Silver, T. Hubert, J. Schrittwieser, I. Antonoglou, M. Lai, A. Guez, M. Lanctot, L. Sifre, D. Kumaran, T. Graepel, T. Lillicrap, K. Simonyan, D. Hassabis, A general reinforcement learning algorithm that masters chess, shogi and Go through self-play, Science 2018, 262, 1140-1144. https://doi.org/10.1126/science.aar6404
[4] A. W. Senior, R. Evans, J. Jumper, J. Kirkpatrick, L. Sifre, T. Green, C. Qin, A. Žídek, A. W. R. Nelson, A. Bridgland, H. Penedones, S. Petersen, K. Simonyan, S. Crossan, P. Kohli, D. T. Jones, D. Silver, K. Kavukcuoglu, D. Hassabis, Improved protein structure prediction using potential from deep learning, Nature 2020, 577, 706-710. https://doi.org/10.1038/s41586-019-1923-7
[5] K. T. Simons, R. Bonneau, I. Ruczinski, D. Baker, Ab initio protein structure prediction of CASPIII targets using ROSETTA, Proteins: Struct. Funct. Genet. Suppl. 1999, 3, 171-176. https://doi.org/10.1002/(SICI)1097-0134(1999)37:3+<171::AID-PROT21>3.0.CO;2-Z
[6] B. Kuhlman, G. Dantas, G. C. Ireton, G. Varani, B. L. Stoddard, D. Baker, Design of a novel globular protein fold with atomic-level accuracy, Science 2003, 302, 1364-1368. https://doi.org/10.1126/science.108942
Selected Publications
Selected Publications by David Baker
- Reconfigurable asymmetric protein assemblies through implicit negative design,
Danny D. Sahtoe, Florian Praetorius, Alexis Courbet, Yang Hsia, Basile I. M. Wicky, Natasha I. Edman, Lauren M. Miller, Bart J. R. Timmermans, Justin Decarreau, Hana M. Morris, Alex Kang, Asim K. Bera, David Baker,
Science 2022.
https://doi.org/10.1126/science.abj7662 - dCas9 fusion to computer-designed PRC2 inhibitor reveals functional TATA box in distal promoter region,
Shiri Levy, Logeshwaran Somasundaram, Infencia Xavier Raj, Diego Ic-Mex, Ashish Phal, Sven Schmidt, Weng I. Ng, Daniel Mar, Justin Decarreau, Nicholas Moss, Ammar Alghadeer, Henrik Honkanen, Jay Sarthy, Nicholas Vitanza, R. David Hawkins, Julie Mathieu, Yuliang Wang, David Baker, Karol Bomsztyk, Hannele Ruohola-Baker,
Cell Rep. 2022, 38, 110457.
https://doi.org/10.1016/j.celrep.2022.110457 - Interpreting neural networks for biological sequences by learning stochastic masks,
Johannes Linder, Alyssa La Fleur, Zibo Chen, Ajasja Ljubetič, David Baker, Sreeram Kannan, Georg Seelig,
Nat. Mach. Intell. 2022, 4, 41–54.
https://doi.org/10.1038/s42256-021-00428-6 - Computational Enzyme Design,
Gert Kiss, Çelebi-Ölçüm, Rocco Moretti, David Baker, K. N. Houk,
Angew. Chem. Int. Ed. 2013, 52, 5700–5725.
https://doi.org/10.1002/anie.201204077 - ROSETTA3: An Object-Oriented Software Suite for the Simulation and Design of Macromolecules,
Andrew Leaver-Fay, Michael Tyka, Steven M. Lewis, Oliver F. Lange, James Thompson, Ron Jacak, Kristian W. Kaufmann,
P. Douglas Renfrew, Colin A. Smith, Will Sheffler, Ian W. Davis, Seth Cooper, Adrien Treuille, Daniel J. Mandell, Florian Richter, Yih-En Andrew Ban, Sarel J. Fleishman, Jacob E. Corn, David E. Kim, Sergey Lyskov, Monica Berrondo, Stuart Mentzer, Zoran Popović, James J. Havranek, John Karanicolas, Rhiju Das, Jens Meiler, Tanja Kortemme, Jeffrey J. Gray, Brian Kuhlman, David Baker, Philip Bradley,
Methods Enzymol. 2011, 487, 545–574.
https://doi.org/10.1016/S0076-6879(11)87019-9 - Quantitative reactivity profiling predicts functional cysteines in proteomes,
Eranthie Weerapana, Chu Wang, Gabriel M. Simon, Florian Richter, Sagar Khare, Myles B. D. Dillon, Daniel A. Bachovchin, Kerri Mowen, David Baker, Benjamin F. Cravatt,
Nature 2010, 468, 790–795.
https://doi.org/10.1038/nature09472 - Kemp elimination catalysts by computational enzyme design,
Daniela Röthlisberger, Olga Khersonsky, Andrew M. Wollacott, Lin Jiang, Jason DeChancie, Jamie Betker, Jasmine L. Gallaher, Eric A. Althoff, Alexandre Zanghellini, Orly Dym, Shira Albeck, Kendall N. Houk, Dan S. Tawfik, David Baker,
Nature 2008, 453, 190–195.
https://doi.org/10.1038/nature06879
Selected Publications by Demis Hassabis
- Magnetic control of tokamak plasmas through deep reinforcement learning,
Jonas Degrave, Federico Felici, Jonas Buchli, Michael Neunert, Brendan Tracey, Francesco Carpanese, Timo Ewalds, Roland Hafner, Abbas Abdolmaleki, Diego de las Casas, Craig Donner, Leslie Fritz, Cristian Galperti, Andrea Huber, James Keeling, Maria Tsimpoukelli, Jackie Kay, Antoine Merle, Jean-Marc Moret, Seb Noury, Federico Pesamosca, David Pfau, Olivier Sauter, Cristian Sommariva, Stefano Coda, Basil Duval, Ambrogio Fasoli, Pushmeet Kohli, Koray Kavukcuoglu, Demis Hassabis, Martin Riedmiller,
Nature 2022, 602, 414–419.
https://doi.org/10.1038/s41586-021-04301-9 - Reimagining chess with AlphaZero,
Nenad Tomašev, Ulrich Paquet, Demis Hassabis, Vladimir Kramnik,
Commun. ACM 2022, 65, 60–66.
https://doi.org/10.1145/3460349 - Mastering the game of Go with deep neural networks and tree search,
David Silver, Aja Huang, Chris J. Maddison, Arthur Guez, Laurent Sifre, George van den Driessche, Julian Schrittwieser, Ioannis Antonoglou, Veda Panneershelvam, Marc Lanctot, Sander Dieleman, Dominik Grewe, John Nham, Nal Kalchbrenner, Ilya Sutskever, Timothy Lillicrap, Madeleine Leach, Koray Kavukcuoglu, Thore Graepel, Demis Hassabis,
Nature 2016, 529, 484–489.
https://doi.org/10.1038/nature16961 - Human-level control through deep reinforcement learning,
Volodymyr Mnih, Koray Kavukcuoglu, David Silver, Andrei A. Rusu, Joel Veness, Marc G. Bellemare, Alex Graves, Martin Riedmiller, Andreas K. Fidjeland, Georg Ostrovski, Stig Petersen, Charles Beattie, Amir Sadik, Ioannis Antonoglou, Helen King, Dharshan Kumaran, Daan Wierstra, Shane Legg, Demis Hassabis,
Nature 2015, 518, 529–533.
https://doi.org/10.1038/nature14236 - Decoding representations of scenes in the medial temporal lobes,
Heidi M. Bonnici, Dharshan Kumaran, Martin J. Chadwick, Nikolaus Weiskopf, Demis Hassabis, Eleanor A. Maguire,
Hippocampus 2011.
https://doi.org/10.1002/hipo.20960
Selected Publications by John Jumper
- Structure of the decoy module of human glycoprotein 2 and uromodulin and its interaction with bacterial adhesin FimH,
Alena Stsiapanava, Chenrui Xu, Shunsuke Nishio, Ling Han, Nao Yamakawa, Marta Carroni, Kathryn Tunyasuvunakool, John Jumper, Daniele de Sanctis, Bin Wu, Luca Jovine,
Nat. Struct. Mol. Biol. 2022, 29, 190–193.
https://doi.org/10.1038/s41594-022-00729-3
Selected Publications by Demis Hassabis and John Jumper
- AlphaFold Protein Structure Database: massively expanding the structural coverage of protein-sequence space with high-accuracy models,
Mihaly Varadi, Stephen Anyango, Mandar Deshpande, Sreenath Nair, Cindy Natassia, Galabina Yordanova, David Yuan, Oana Stroe, Gemma Wood, Agata Laydon, Augustin Žídek, Tim Green, Kathryn Tunyasuvunakool, Stig Petersen, John Jumper, Ellen Clancy, Richard Green, Ankur Vora, Mira Lutfi, Michael Figurnov, Andrew Cowie, Nicole Hobbs, Pushmeet Kohli, Gerard Kleywegt, Ewan Birney, Demis Hassabis, Sameer Velankar,
Nucleic Acids Res. 2022, 50, D439–D444.
https://doi.org/10.1093/nar/gkab1061 - Protein structure predictions to atomic accuracy with AlphaFold,
John Jumper, Demis Hassabis,
Nat. Methods 2022, 19, 11–12.
https://doi.org/10.1038/s41592-021-01362-6 - Highly accurate protein structure prediction for the human proteome,
Kathryn Tunyasuvunakool, Jonas Adler, Zachary Wu, Tim Green, Michal Zielinski, Augustin Žídek, Alex Bridgland, Andrew Cowie, Clemens Meyer, Agata Laydon, Sameer Velankar, Gerard J. Kleywegt, Alex Bateman, Richard Evans, Alexander Pritzel, Michael Figurnov, Olaf Ronneberger, Russ Bates, Simon A. A. Kohl, Anna Potapenko, Andrew J. Ballard, Bernardino Romera-Paredes, Stanislav Nikolov, Rishub Jain, Ellen Clancy, David Reiman, Stig Petersen, Andrew W. Senior, Koray Kavukcuoglu, Ewan Birney, Pushmeet Kohli, John Jumper, Demis Hassabis,
Nature 2021, 596, 590–596.
https://doi.org/10.1038/s41586-021-03828-1 - Highly accurate protein structure prediction with AlphaFold,
John Jumper, Richard Evans, Alexander Pritzel, Tim Green, Michael Figurnov, Olaf Ronneberger, Kathryn Tunyasuvunakool, Russ Bates, Augustin Žídek, Anna Potapenko, Alex Bridgland, Clemens Meyer, Simon A. A. Kohl, Andrew J. Ballard, Andrew Cowie, Bernardino Romera-Paredes, Stanislav Nikolov, Rishub Jain, Jonas Adler, Trevor Back, Stig Petersen, David Reiman, Ellen Clancy, Michal Zielinski, Martin Steinegger, Michalina Pacholska, Tamas Berghammer, Sebastian Bodenstein, David Silver, Oriol Vinyals, Andrew W. Senior, Koray Kavukcuoglu, Pushmeet Kohli, Demis Hassabis,
Nature 2021, 596, 583–589.
https://doi.org/10.1038/s41586-021-03819-2 - Applying and improving AlphaFold at CASP14,
John Jumper, Richard Evans, Alexander Pritzel, Tim Green, Michael Figurnov, Olaf Ronneberger, Kathryn Tunyasuvunakool, Russ Bates, Augustin Žídek, Anna Potapenko, Alex Bridgland, Clemens Meyer, Simon A. A. Kohl, Andrew J. Ballard, Andrew Cowie, Bernardino Romera-Paredes, Stanislav Nikolov, Rishub Jain, Jonas Adler, Trevor Back, Stig Petersen, David Reiman, Ellen Clancy, Michal Zielinski, Martin Steinegger, Michalina Pacholska, Tamas Berghammer, David Silver, Oriol Vinyals, Andrew W. Senior, Koray Kavukcuoglu, Pushmeet Kohli, Demis Hassabis,
Proteomics 2021.
https://doi.org/10.1002/prot.26257 - Improved protein structure prediction using potentials from deep learning,
Andrew W. Senior, Richard Evans, John Jumper, James Kirkpatrick, Laurent Sifre, Tim Green, Chongli Qin, Augustin Žídek, Alexander W. R. Nelson, Alex Bridgland, Hugo Penedones, Stig Petersen, Karen Simonyan, Steve Crossan, Pushmeet Kohli, David T. Jones, David Silver, Koray Kavukcuoglu, Demis Hassabis,
Nature 2020, 577, 706–710.
https://doi.org/10.1038/s41586-019-1923-7
Also of Interest
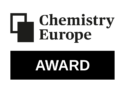
The 10.000 EUR award recognizes outstanding contributions to the field of theoretical/computational chemistry with an optional focus on AI in chemistry
- Nobel Prize in Chemistry 2023,
ChemistryViews 2023.
Moungi Bawendi, Louis Brus, and Alexei Ekimov were honored for “the discovery and synthesis of quantum dots” - Collection: Nobel Prize in Chemistry
Collection of interviews with Nobel Laureates and Nobel Prize quizzes