Professor Alexei Lapkin is the Director of the Innovation Centre in Digital Molecular Technologies (iDMT) at the University of Cambridge, UK. It is an incubator supporting companies in the transformation of chemistry into the digital domain.
Here, he and Dr. Vera Koester talk for ChemistryViews about the work of the center, the state of the art and challenges in transforming chemistry into the digital realm, and what many new things chemists have to/should be dealing with that they haven’t faced before.
Can you explain what the iDMT does?
We are interested in anything to do with making molecules in terms of digitalization. We are trying to help companies who are involved in making molecules or discovering molecules to do it more efficiently using the emerging tools. These are mostly digital and include access to data, how to manage data, how to run experiments using robotic equipment, how to link all of the workflows in an environment that is pretty much all connected into almost like an Internet of Things-type environment.
So you can imagine laboratories where routine experiments can be given to a robot to do, where analysis can be done by machines that are automated. The results can then feed into the next workflow, which may involve machine learning to analyze data for understanding the trends, for example, or they can feed into a workflow and process development.
This means a lab with different interconnected devices that exchange data? What do you use it for?
One lab is dedicated to high-throughput equipment and high-throughput-type workflows. When scientists are interested in generating libraries of molecules to understand reactivity patterns, to develop machine learning models, but there is no data in the literature, they need to generate the data very quickly. So for that reason, they use high-throughput techniques. If you use high throughput in synthesis, you have to use high throughput analysis, and that generates a lot of data. So you need to figure out how to deal with these amounts of data.
It is quite a tricky thing to design the initial high-throughput experiments because it is a logistical problem of how many bottles you have on feeds, where you put them, how you access which combinations, and so on. You have to have some kind of software to do that; manually it is not possible. Also, you cannot really manually analyze all of this data. So you need to know how to label everything, how to transfer it into a database, etc.
You can imagine that chemists who have studied traditional organic chemistry would struggle to run this. So we are making sure that chemists, in industry particularly, are informed about what is available, who the vendors are, who provides these solutions, and what the benefits are.
Some time ago, programs emerged that could predict synthesis pathways for specific molecules used in the pharmaceutical industry, for example. Do you use them as well?
Yes, we use them as well. The goal of the center is to help companies adopt these techniques and take advantage of them. So we give them access to everything that’s out there.
So you are an expert in all these areas and pass on your knowledge to others?
Yes, exactly. We have academics in our center who are developing these kinds of codes, who are developing these kinds of retrosynthesis or forward synthesis prediction tools, or scientists who are interested in, let’s say, predictive toxicology, or my group is interested in circular economy, that is, how to use molecular structures in a circular way and how to make sure that you get the right structures from waste materials and so on. These are all algorithms.
We basically show companies how these things work, and they think about what they can do with them. So it is kind of a melting pot where everyone from end users in the pharmaceutical industry, to academics, to small companies providing services, and companies involved in chemical discovery or product development come together.
Based on your experience, what do you think the laboratory of the near future will look like?
Well, in the near future, the lab will not necessarily be that much different from advanced synthetic labs, but it will be more equipped with machines. So we do not have round-bottom flasks in the lab, and instead, we do experiments with robots. You set up these experiments and then they are done automatically.
This is done to improve reproducibility and to make sure that the lab can work 24/7 to save time. There are also lots of other reasons why you would want to give some of the experiments to machines. For example, to make sure that people are not around when dangerous reactions occur.
What are some challenges besides learning how to best handle these machines?
Challenges in this field are especially found in discovery chemistry, where accidental observations are important. It is a challenge for this field to make sure we do not lose that. So there is a subcommunity here in this field that is looking at discovery chemistry with machines and how to make sure that there is serendipity there.
So that the machine learns to make mistakes?
Well, the machine will not think that it is an error. There are “mistakes” that are programmed.
Humans are very good at spotting unusual things, and that is typically how we make discoveries. So we need algorithms to do the same thing.
Let’s say I’m an organic chemist. How do I start using these new tools?
Digital labs are not yet ubiquitous. They are beginning to be developed. The most advanced labs are in big pharma that have fantastic facilities for high throughput and machine learning, etc., but even these are still growing and developing.
Universities do not have that many resources. Therefore, it is important to make sure that digital skills are incorporated into undergraduate and postgraduate curricula so that chemists know what they can access and how.
And when I would come to your lab?
As a first step, synthetic chemists, for example, who come to work with me, are encouraged not to rush with doing experiments in a flask on the first day. I ask them to first check what retrosynthesis and machine learning say, to do data mining for their chemistry. Once they have done all these things with these tools — and they have never seen them before — only then, when their knowledge has been enhanced by these tools and they have more things to think about, will their experience in the lab be enhanced.
That is a different approach, so to speak. They need to know what things are available and they need to know the limitations, know what you can do and what you cannot do. It is the same as when you use an NMR spectrometer, for example. You need to know what you have measured correctly.
This approach generates a lot of data. Does that mean that the person with the most data has a big advantage?
Yeah, this is the biggest challenge and the biggest pain in this field in chemistry because we have all these very large data sets that are non-public like Sci-Finder or Reaxys, for example. The data is locked behind rather expensive paywalls. Big corporations have internal data sets that are extremely valuable and they will never share them. There are, however, approaches to more open data sources, such as releasing certain data sets for certain applications, for example, to test new codes.
Some companies are beginning to share data specifically for machine-learning purposes. That was a case of several pharma companies releasing specific data sets of molecules like failed drug molecules or some other projects. Those are actually very valuable for the community that does machine learning to sort of reuse the molecules for other purposes.
There are several projects globally aimed at creating open data sets in chemistry. There is PubMed for MedChem data or ChemSpider, the Royal Society of Chemistry dataset. There is an open chemistry data project based at MIT in the US, the Chemistry Implementation Network (ChIN), and another big one in Germany, called NFDI4Chem.
What do you use at iDMT?
We use everything we can get open access to and where our existing licenses do not prevent us from sharing information within internal projects. Obviously, we use PubMed and all of the open-source databases, but we also have a research agreement so we can datamine Reaxys.
You also need lots of computing power?
Not all of the chemistry problems are very large. When you go to drug discovery and proteins, of course, they’re the big models, which take a lot of resources. That’s where Google and others are involved and DeepMind. Those models take a lot of time to train.
We mostly use relatively modest computational resources. Sometimes we go to Google Colab or AWS (Amazon Web Services).
However, we need to be a little bit careful, and there is increasing discussion about being a little bit clever about what to do, and what to avoid, so as not to run computers for trivial tasks.
What about the codes? Are they free?
It’s a very collaborative field and in computer science, there is a spirit that all the codes are shared. So every code is open-source. At least in academic software, everything is open-source. We don’t publish anything in journals unless there is an open code attached because that’s the standard.
Do many journals offer this?
It’s more of a requirement of the community. The standard journals don’t know this, but they begin to publish more and more papers with machine learning.
I’m an associate editor for the Chem. Eng. Journal, and that’s not a traditional computational journal. However, we get more and more papers about machine learning. As an editor, I reject all papers that don’t have a GitHub, so a link to the code. If they do not have a link to the code the paper doesn’t even get sent to reviewers. They have to have it.
I understand that you’re cooperating with SMEs. So if I’m an SME and interested in adopting such processes, could I try to collaborate with you?
Yes, we are open to working with any company and also if you are a big company. We are in the process of expanding the network of companies we can work with.
Is this opportunity well received by the companies or is there still a lack of knowledge and acceptance?
It depends. The types of companies we work with, so far, include hardware vendors and software vendors, chemical companies for the manufacture of molecules, and also a little bit on medicinal discovery. The companies for whom it was the easiest to see the benefit are probably hardware vendors. They see that they need to adapt their software to be ready for digital labs. The same is true for machine-learning software vendors. They need to tweak their software for specific workflows in chemistry and pharma.
For chemical companies, SMEs, it is quite often a lack of resources and internal skills. So we now have one slightly larger SME in the UK. They had their own sort of digitalization plan but actually, they have no skills inside the company at all and so we had to work with them to get them to the point when they actually can start getting benefits. This means developing projects where they can learn as well as where there’s going to be some external funding from the government to hire a person to get trained. These sorts of things.
We work with each company independently to figure out what is it they need and how to get them the result they want.
And if I’m an academic and would like to make use of these technologies?
That’s completely fine. We normally ask academics to then also be part of the center and also talk to the SMEs and the big companies and the people who are in the center so that there is some sharing of expertise.
We have some equipment that academics want to use, but we also have access to the industry to get insight into the market pool, and find out what they want. So we have a bunch of people working at the center. I would say that not everyone knows how to exploit us very well yet, but it is developing.
It must be exciting to work in such a new field. What trends would you like to see develop in the near future?
First of all, the undergraduate chemistry curricula have to be changed. Students must be equipped with mathematics and computer skills. I don’t accept the statement that you can’t teach math to organic chemists. That’s just wrong. I studied chemistry with two years of maths in it. So if I can do it, anyone can do it.
Second, once you go into this digital world the boundaries more or less disappear. You need to have your core skills, of course, in the area of chemistry you work in but then in the labs, the traditional boundaries of disciplines are kind of disappearing. It is getting a lot fuzzier, which I like. In developing experiments for, let’s say, high throughput, synthetic chemists need to understand a lot more of physical chemistry and a little bit of chemical engineering. They start working with reactors and suddenly they have to think about boiling points, pressure, precipitation, and all sorts of things, which is chemical engineering.
The iDMT and also our Centre for Doctoral Training ‘SynTech’, are all interdisciplinary. All students there are together and that’s the absolute necessity for this area.
What got you initially interested in machine learning?
I guess I was lucky. I was working in chemical engineering at Warwick University, and next to us was a center for complexity mathematics, which is basically machine learning. They were looking for end users to co-supervise their Ph.D. students. So we started discussing the challenges of sort of tricky chemistry when you run reactions in condensed phases or multi-phase systems. You quite often don’t have all of the information, so rigorous chemical engineering is not really possible. Most processes in pharma are not optimized because it is really hard to learn enough about them to develop rigorous process models.
We started talking to mathematicians about how to conclude what you don’t know. How to use data to infer what’s happening, and how to use statistics. Out of that came machine learning.
Can you say a bit more about your research? You said you’re working on circularity?
My group is called sustainable reaction engineering. We’re interested in how to make molecules most sustainably. Sometimes this means not making them because sustainability is more than just chemistry.
The feedstocks are a big topic because the sourcing of molecules in a non-circular way is one of the biggest contributions to global climate change. This is a mathematical challenge. Of course, you have chemistry problems there, but also it’s a network of reactions and of molecules, and networks are a mathematical field. So we deal with networks to figure out what the optimal structure of the network is, or basically the supply chain of molecules.
In addition, we also work with the process industry on specific algorithms for faster process development or, let’s say, automated experiments, that is, how to run experiments where it isn’t a human that makes a decision in an experiment but the machine. So this sort of intelligent design of experiments based on the hypothesis generated by algorithms and then making the results interpretable to humans so that you can actually see why the machine is doing this and what’s the output. We do this mostly in collaboration with mathematicians.
What do you enjoy most about your research?
The students. This is of course the main enjoyment. I mean I’ve done my bit before, but now it’s primarily seeing them grow and develop, and they’re all much smarter than me. It’s just giving them a bit of guidance and motivating them and showing them what they can deliver. That’s really the best thing.
Do you see any problems generated by this field?
It’s a very big field and it’s also developing quickly. There are some underlying kind of problems in the field. People are jumping on the band wagon and starting to use random algorithms to do stuff and then trying to publish the results which, of course, is not right because people don’t even know what they’re doing. I can’t claim that I know everything I’m doing, but it’s always a necessity to keep rigor like in any field.
It’s a hype to use machine learning for everything. We need to wait for the hype to go down a little bit so that the sensible stuff will remain. Then it will actually be much more useful.
Which new business models are emerging?
There are quite a few. First of all, there were startups on using large data to do retrosynthesis. Now several of them have products on the market already successful.
Some companies are looking at optimizing the chemicals they use to make sure that people don’t have enormous inventories that they don’t need. Large companies, of course, are looking at decarbonization and the opportunities that come from different chemicals, different energy sources, different feedstocks, as well as optimizing those things. So there are quite a few things that are potentially possible.
Digitalization allows us to map everything. Once you have the mapping, once you have the data, you can do all sorts of analysis and then optimizations and synergies and so on. The problem in chemistry was for a long time that it’s an extremely opaque market. Nobody knows where what is and how, and it is almost impossible to buy on an open market, you have to go through some intermediate companies. I think this will change and many unnecessary expenses will also disappear.
Chemists are proud when they synthesize a molecule, for example. That seems a bit old-fashioned now, because now maybe you can be proud of how many molecules you’ve synthesized, or maybe it’s not even you who synthesizes them anymore?
It’s a philosophical discussion. You can have an algorithm designing new molecules and synthesizing them. Then who has discovered it? The person who wrote the algorithm, who actually doesn’t know anything about chemistry? Yeah, that’s the reality, it will happen.
The reason that’s going to happen is that the best synthetic chemists have an enormous knowledge of facts, and can connect them in their minds and figure out relationships and interesting interactions. That’s pretty much what machine learning does, right? It does exactly that when there’s enough data. In chemistry, there’s just not enough data to make that work. So the focus is on data generation and models that work with relatively small amounts of data. So you still can’t replace the synthetic chemist or any chemist because it’s not the same as in areas where you have a lot of continuous signals like images. In image analysis, algorithms are way superior to humans but in chemistry, that’s not necessarily the case.
This then also changes the way we talk about chemistry or how we present our results.
Yeah, this will change. We’re already beginning to advocate for academic papers having digitally encoded data. That is absolutely necessary because there are so many scraping algorithms. I can scrape journals — well I can’t, but in principle, it’s free software, I can take it and scrape journals and get all the data that we need. We don’t need papers, we just need access to the webpage of the journal and then we just scrape it and put it in our database. I don’t even need to know who’s done it. It just becomes a record somewhere in the database. This is definitely a side of the future that needs to be thought about in terms of how we deal with it.
Then how can you be sure that the data is correct or if something is wrong?
When you have robots, you basically publish the protocol and then try it out on your robot.
Okay, so you would need another robot to do the peer review.
Thanks for the insight into this exciting field and for sharing your thoughts on the future.
Alexei Lapkin studied biochemistry at Novosibirsk State University, Russia. He then worked at the Boreskov Institute of Catalysis in Novosibirsk before moving to the University of Bath, UK, where he was a research fellow and obtained his Ph.D. in multiphase membrane catalysis in 2000.
He was a Lecturer at the University of Bath, UK, until 2009, and a Professor at the University of Warwick until 2013. Since 2013, Alexei Lapkin has been a Professor of Sustainable Reaction Engineering at the Department of Chemical Engineering and Biotechnology, University of Cambridge, UK, and Director of the Innovation Centre in Digital Molecular Technologies (iDMT) at the University of Cambridge.
Selected Publications
- J. Raphael Seidenberg, Ahmad A. Khan, Alexei A. Lapkin, Boosting autonomous process design and intensification with formalised domain knowledge, Comp. Chem. Engng. 2023, 169, 108097. https://doi.org/10.1016/j.compchemeng.2022.108097
- Daniel S. Wigh, Jonathan M. Goodman, Alexei A. Lapkin, A review of molecular representation in the age of machine learning, WIREs Comp. Mol. Sci. 2022, e1603. https://doi.org/10.1002/wcms.1603
- Zhimian Hao, Magda H. Barecka, Alexei A. Lapkin, Accelerating net zero from the perspective of optimising a carbon capture and utilisation system, Energy Environ. Sci. 2022, 15, 2139-2153. https://doi.org/10.1039/D1EE03923G
- Jana M. Weber, Zhen Guo, Alexei A. Lapkin, Discovering circular process solutions through automated reaction network optimisation, ACS Engineering Au 2022, 2, 333-349. https://doi.org/10.1021/acsengineeringau.2c00002
- Mohammed I. Jeraal, Simon Sung, Alexei A. Lapkin, A Machine Learning-Enabled Autonomous Flow Chemistry Platform for Process Optimization of Multiple Reaction Metrics, Chemistry–Methods 2021, 2, 71-77. https://doi.org/10.1002/cmtd.202000044
- Magda H. Barecka, Joel W. Ager, Alexei A. Lapkin, Economically viable CO2 electro reduction embedded within ethylene oxide manufacturing, Energy Env. Sci., 14 (2021) 1530-1543. https://doi.org/10.1039/D0EE03310C
- Kobi C. Felton, Jan G. Rittig, Alexei A. Lapkin, Summit: Benchmarking machine learning methods for reaction optimisation, Chemistry-Methods 2021, 1, 116-122. https://doi.org/10.1002/cmtd.202000051
- Jana M. Weber, Constantin P. Lindenmeyer, Pietro Liò, Alexei A. Lapkin, Teaching sustainability as complex systems approach: a sustainable development goals workshop, Int. J. Sustain. Edu. 2021, 22:8, 25-41. https://doi.org/10.1108/IJSHE-06-2020-0209
- Jana M. Weber, Zhen Guo, Chonghuan Zhang, Artur M. Schweidtmann, Alexei A. Lapkin, Chemical data intelligence for sustainable chemistry, Chem. Soc. Rev. 2021. https://doi.org/10.1039/d1cs00477h
- Peter Fantke, Claudio Cinquemani, Polina Yaseneva, Jonathas De Mello, Henning Schwabe, Bjoern Ebeling, Alexei A. Lapkin, Transition to sustainable chemistry through digitalization, Chem 2021. https://doi.org/10.1016/j.chempr.2021.09.012
Also of Interest
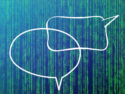
Discussing science communication, AI in chemistry, publication ethics, and the purpose of life with an AI